

One of Kish's introductions for "Gangsta Bitch" and Apache's about to tell you what every man wants. One of Kish's introductions for "What A Thug About"
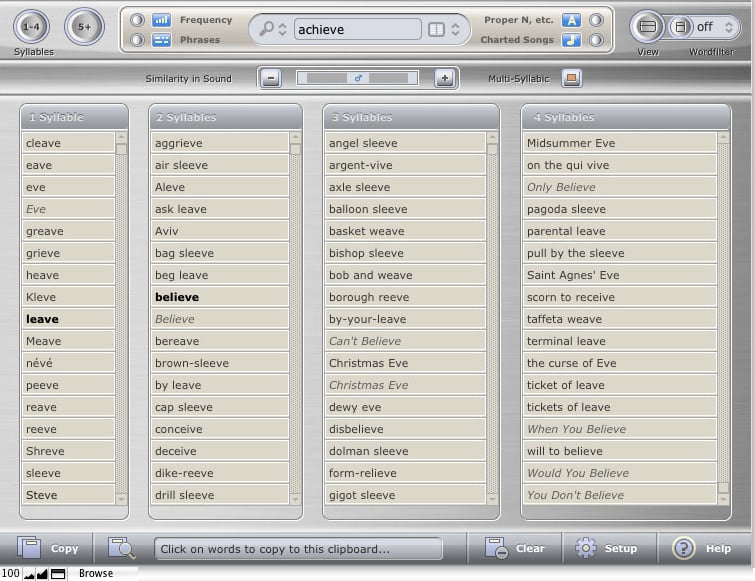
If you've ever listened in to Veteran Child on Gen X, I think it's safe to say people don't know shit about what a thug about.
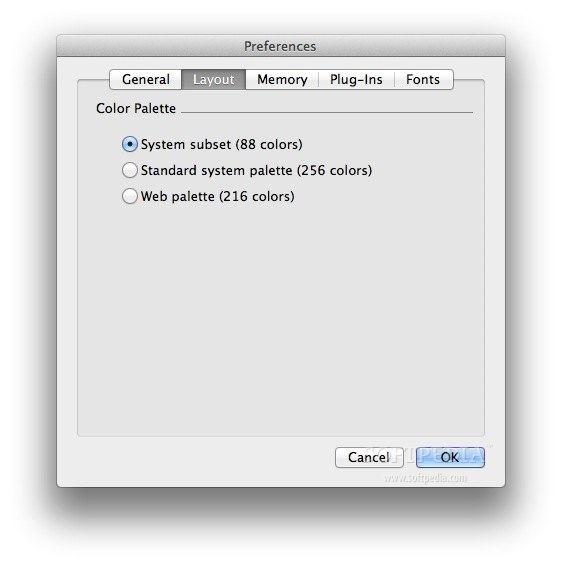
This is a shout out to the lyrics of Kish's one-hit wonder 'I Rhyme the World in 80 Days' The caller said "Africa", Kish corrected him saying "No, Japan". During a caller request, Kish said it was time to go back to the homeland.The DJ in Saints Row 2 is Kish, voiced by Andrew Kishino, who is also the voice actor for Donnie. Some Los Carnales vehicles are set to this station.Eddie Francis is a real radio DJ that works for KUBE in Seattle.Masta Killa featuring ODB & RZA - Old Man.Aisha - Don't Fuck Me Like I'm Your Wife., how well they go with each other when played simultaneously.Eddie Francis is the DJ for KRhyme in Saints Row. This task is challenging because it is difficult to formulate hand-crafted rules or construct a large labeled dataset to perform supervised learning. Our method uses self-supervised and joint-embedding techniques for estimating vocal-accompaniment compatibility. We train vocal and accompaniment encoders to learn a joint-embedding space of vocal and accompaniment tracks, where the embedded feature vectors of a compatible pair of vocal and accompaniment tracks lie close to each other and those of an incompatible pair lie far from each other. To address the lack of large labeled datasets consisting of compatible and incompatible pairs of vocal and accompaniment tracks, we propose generating such a dataset from songs using singing voice separation techniques, with which songs are separated into pairs of vocal and accompaniment tracks, and then original pairs are assumed to be compatible, and other random pairs are not. We achieved this training by constructing a large dataset containing 910,803 songs and evaluated the effectiveness of our method using ranking-based evaluation methods.Ī music mashup combines audio elements from two or more songs to create a new work. To reduce the time and effort required to make them, researchers have developed algorithms that predict the compatibility of audio elements. Prior work has focused on mixing unaltered excerpts, but advances in source separation enable the creation of mashups from isolated stems (e.g., vocals, drums, bass, etc.). In this work, we take advantage of separated stems not just for creating mashups, but for training a model that predicts the mutual compatibility of groups of excerpts, using self-supervised and semi-supervised methods. Specifically, we first produce a random mashup creation pipeline that combines stem tracks obtained via source separation, with key and tempo automatically adjusted to match, since these are prerequisites for high-quality mashups. To train a model to predict compatibility, we use stem tracks obtained from the same song as positive examples, and random combinations of stems with key and/or tempo unadjusted as negative examples. To improve the model and use more data, we also train on "average" examples: random combinations with matching key and tempo, where we treat them as unlabeled data as their true compatibility is unknown. To determine whether the combined signal or the set of stem signals is more indicative of the quality of the result, we experiment on two model architectures and train them using semi-supervised learning technique. Finally, we conduct objective and subjective evaluations of the system, comparing them to a standard rule-based system. This article employs Stuart Hall’s concept of ‘articulation’ to show how, in the mid-2000s, a loose coalition of tech activists and commentators worked to position mashup music as ‘the sound of the Internet’.
